Can AI effectively predict flooding?
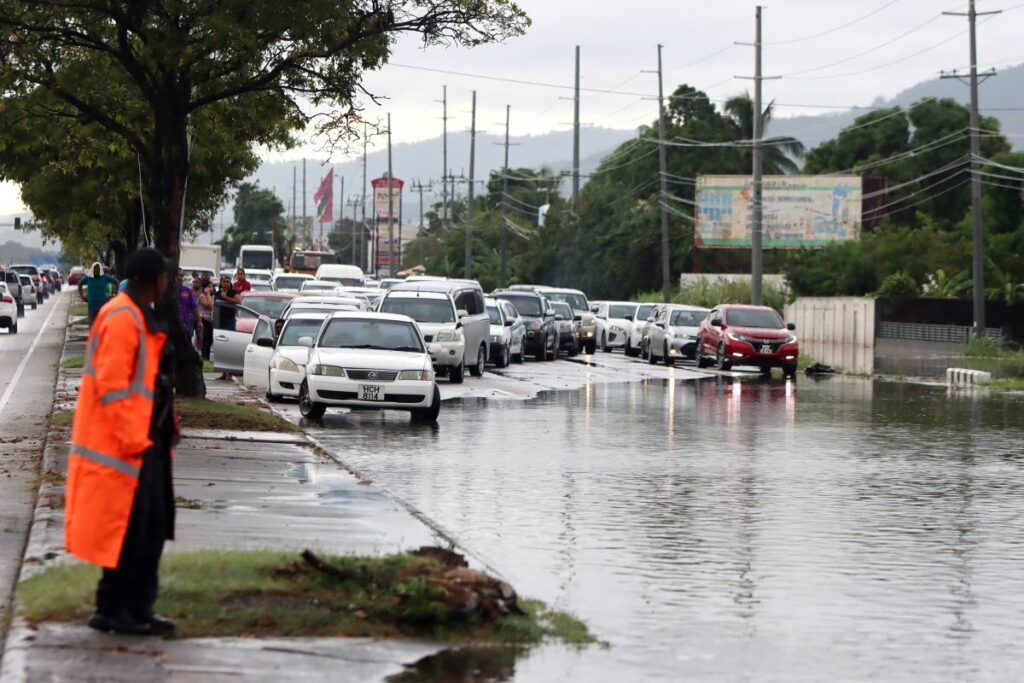
BRENDON JAMES
Artificial intelligence (AI) subtly yet significantly influences our daily digital interactions. From the tailored news feeds on our social media platforms to the eerily accurate friend suggestions and targeted advertisements, AI's footprint is undeniable.
The advent of generative AI, exemplified by innovations such as ChatGPT, has brought the commercial potential of AI to the forefront.
Developed by OpenAI, ChatGPT is part of a broader category of technology known as large language models (LLMs), which are designed to understand, generate and interact with human language in a way that's both comprehensive and contextually relevant.
This evolution of AI from a behind-the-scenes actor to a headline-grabbing phenomenon raises critical questions about its application beyond the digital entertainment and advertising realms, particularly in addressing the pressing challenges of the real economy.
As professionals in the energy sector, we find ourselves at the forefront of combating one of the most daunting challenges of our era – climate change.
The imperative for climate adaptation has never been more urgent, with the increasing frequency of extreme weather events highlighting the vulnerability of our communities and economies.
Climate adaptation involves developing and implementing strategies to mitigate the impacts of climate change, from enhancing infrastructure resilience to adopting sustainable agricultural practices.
Challenge of flooding
Trinidad and Tobago, like many island nations, faces significant risks from flooding. This is exacerbated by its geographical location, which makes it prone to heavy rainfall events, particularly during the hurricane season. The country has experienced several devastating floods in recent years, affecting thousands of lives and causing substantial economic damage. The need for effective flood prediction and management strategies is therefore critical.
This leads to the hypothesis: Can AI effectively predict flooding in Trinidad and Tobago?
To explore this, I embarked on a project using the current open-source machine learning (ML) and AI tools, armed with nothing but a laptop and a healthy dose of curiosity.
The journey began with the search for relevant data, a quest that led me to a treasure trove of weather data spanning over a decade.
Using Beautiful Soup, a Python library for web scraping, I compiled five years of weather data from 2019-2023, encompassing rainfall, temperature and cloud coverage.
Identifying reliable data on flooding events posed a significant challenge because of the scarcity of public records. However, by exploring resources such as the Meteorological Office, TT Weather Centre, Relief Web and the Caribbean Catastrophe Risk Insurance Facility, I managed to gather comprehensive flooding data for the same period. The data frame build identified 2,266 flooding events over the period in question.
In my quest to understand the evolving nature of flooding, I embarked on a detailed analysis without presuppositions.
The initial phase focused on quantifying the extent of flooding over recent years, facilitated by a comparative analysis of rainfall and flooding events between 2019 and 2022.
Observations from 2019-2022
A clear upward trajectory in the number of flooding events was observed, punctuated by a slight reduction in 2023.
This trend underscores the growing challenge of flooding in the region, necessitating advanced predictive and management strategies.
Application of AI: Predicting the future
To transition from mere analysis to actionable foresight, we employed TensorFlow, a cutting-edge deep-learning algorithm developed by Google Brain.
TensorFlow's versatility in numerical computation and its robust ML framework facilitated the development of a model capable of learning from five years of data to forecast flooding events for the 2024 rainy season.
Figure four showcases the top 25 areas at risk, highlighting the predicted frequency of flooding events by month and region for enhanced visibility. The model has over 200 areas built in that can be displayed.
Key predictions for 2024
– Peak risk months: May, June, July and September are identified as high-risk periods, with widespread geographic impact anticipated. Notably, areas along the East-West Corridor and Southern Trinidad are expected to face the severest challenges, with Central and Northwest Trinidad also being significant concern points in June.
– Rainfall patterns: Despite an overall expected decrease in monthly rainfall, the intensity of rain (measured as the total inches over a short duration) may rise. This indicates a shift towards more intense, albeit brief, rainfall events, necessitating further detailed analysis for conclusive insights.
– Comparison to previous years: The anticipated flooding this year is predicted to mirror the experiences of 2023 closely, indicating a persistence of the recent trend without significant deviation.
This is just a sample of the insights.
Implications and future directions
This analysis not only highlights the escalating frequency of flooding, but also showcases the power of AI in crafting predictive models that can inform better preparedness and response strategies.
As we look towards the future, it becomes increasingly clear that leveraging such technologies in climate-adaptation efforts is not just beneficial but essential for mitigating the impacts of such natural disasters.
Embracing AI for climate resilience
As we stand at the intersection of technology and environmental stewardship, the findings from this exploration into using AI for flood prediction in TT offer both a caution and a beacon of hope.
The increasing trend in flooding events, as uncovered in our analysis, signals a clear and present danger to our communities, economy and environment.
However, the successful application of AI, through the TensorFlow deep learning framework, illuminates a path forward.
The predictive insights for the 2024 rainy season, identifying specific high-risk periods and areas, are a testament to the potential of AI in transforming our approach to disaster preparedness and response. By harnessing the power of machine learning, we can move from reactive measures to proactive, data-driven strategies that safeguard lives and livelihoods.
What next?
The journey doesn't end here. Building on the foundation laid by this project, the next steps involve:
– Enhancing data collection and analysis: To refine the predictive accuracy of our models, we must invest in more comprehensive and real-time data collection, encompassing a broader range of environmental and socio-economic factors.
– Create a database of GPS locations of flooded areas: We should have a data set of GPS co-ordinates for all towns, villages and boroughs that can allow flooding to be mapped and displayed with this data for better visualisation and analysis.
– Collaborative efforts: Engaging with government agencies, community organisations and international bodies to share insights, resources and strategies for flood mitigation and climate adaptation.
– Public awareness and education: Empowering communities with knowledge and tools for resilience, ensuring that everyone understands the risks and the measures they can take to protect themselves and their properties.
– Policy and infrastructure development: Informing policy decisions and infrastructure projects with AI-driven insights, focusing on sustainable development and climate resilience.
As we advance, the integration of AI in addressing the challenges posed by climate change and natural disasters represents a promising frontier.
This project is a step towards a future where technology and human ingenuity converge to create a safer, more resilient world for all.
Let us embrace this challenge with open arms and collaborative spirits, for the welfare of TT and beyond.
Brendon James is a sustainable energy and risk management professional who has worked in the energy industry for more than 20 years.
His experience spans the entire energy value chain, as he has spent time working in the upstream, downstream, and regulatory aspects of the business.
This article was submitted by the Geological Society of TT (GSTT).
Comments
"Can AI effectively predict flooding?"