Using big data, AI to optimise Trinidad and Tobago’s energy sector
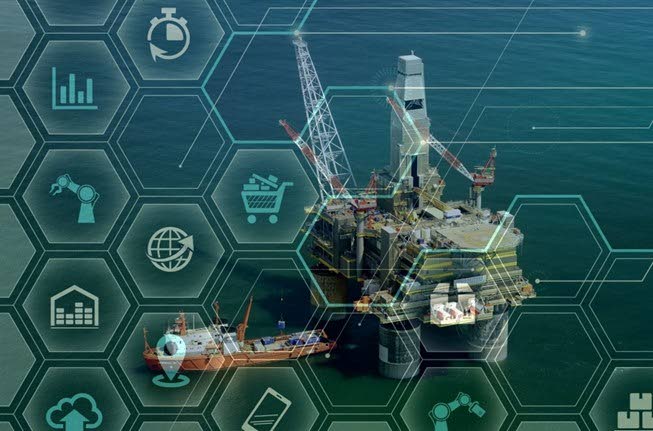
MARISA BAIRACHI
Data analytics and big data have emerged as powerful tools that can revolutionise industries globally.
Data analytics can play a critical role in revitalising mature oil fields in TT. As many of the oil fields in the country have been in production for several decades, we are facing declining production rates, increased operational challenges, fluctuating oil prices, and environmental concerns.
AI is becoming a household name, mostly thanks to the newest generative AI platforms and apps such as ChatGPT, Bard, and DALL-E.
The pace of AI’s evolution calls for swift action to ensure that we are ready to leverage promptly its latest capabilities but also to correctly govern it to deliver trusted digital solutions at scale to the energy industry. The open subsurface data universe (OSDU) and data analytics together have the potential to revolutionise oil and gas exploration by providing a standardised platform for data sharing, integration, and advanced analytics. OSDU is an industry-wide initiative aimed at creating a common data platform for the oil and gas industry, enabling seamless collaboration and data-driven decision-making. The OSDU and data analytics can work together to improve oil and gas exploration and brown field revitalisation.
Data analytics for exploration and production
By analysing seismic data, well logs, and geological information, data analytics can pinpoint potential drilling locations with higher success rates.
Machine learning algorithms can process vast amounts of data to identify promising reservoirs, enabling more targeted and cost-effective exploration efforts.
The OSDU platform provides a standardised data model that allows various data types from different sources to be integrated seamlessly. It enables the consolidation of geophysical data, well logs, seismic surveys, production data, and reservoir models from multiple operators, service companies, and government agencies into a single, unified data repository.
Data analytics can then be applied to this integrated dataset to gain a holistic understanding of the subsurface. By leveraging machine learning algorithms and data visualisation tools, geoscientists and engineers can analyse and interpret the data more effectively, identifying geological structures, hydrocarbon prospects, and exploration opportunities with greater accuracy through predictive modelling, and real-time data analytics.
Furthermore, data analytics can optimise production operations by continuously monitoring well performance, reservoir behaviour, and flow rates.
Predictive maintenance can detect equipment anomalies early, leading to reduced downtime and increased asset reliability. Integrating data analytics into real-time production management enables operators to make well-informed decisions, optimise production rates, and adapt to changing market conditions swiftly.
Data analytics in brown field optimisation
Data analytics plays a crucial role in revitalising mature oil fields, which are characterised by declining production rates and diminishing reservoir pressures.
By harnessing advanced data analytics techniques, operators can optimise production, reduce operational costs, and extend the productive life of these fields.
Here are more details on how data analytics can be applied for the revitalization of mature oil fields:
– Production optimisation: Data analytics allows operators to analyse historical production data and real-time sensor readings from wells and reservoirs. By identifying production trends and performance patterns, data analytics can pinpoint the most productive zones within the reservoir. This information enables operators to optimise well placement and control production rates effectively. Furthermore, machine learning algorithms can process large datasets to identify factors affecting production performance, such as water influx, sand production, or changes in reservoir pressure. Armed with this insight, operators can implement targeted solutions to mitigate production challenges and enhance well performance.
– Predictive maintenance: One of the critical challenges in mature oil fields is equipment reliability. Ageing infrastructure - including pumps, compressors, and pipelines - is prone to frequent breakdowns, leading to costly downtime. Data analytics enables predictive maintenance, where real-time sensor data and historical maintenance records are analysed to predict equipment failures before they occur. Predictive maintenance models leverage machine learning algorithms to detect early signs of equipment degradation or anomalies, alerting operators to take proactive maintenance actions. By preventing unplanned equipment failures, operators can minimise production downtime and optimise maintenance schedules, thereby maximising production uptime.
– Reservoir management and enhanced oil recovery: Data analytics is instrumental in optimising reservoir management in mature oil fields. By integrating real-time data from reservoir sensors and historical production data, operators can build accurate reservoir models. These models allow for better visualisation and understanding of reservoir behaviour, fluid movements, and remaining hydrocarbon reserves. With an accurate reservoir model, operators can implement enhanced oil recovery (EOR) techniques, such as water flooding, gas injection, or chemical injection, to increase hydrocarbon recovery. Data analytics can help identify suitable candidates for EOR and optimise injection rates to maximise oil production.
– Well intervention planning: Well intervention activities, such as workovers or re-completions, are common strategies used to enhance production from mature wells. Data analytics aids in determining the most effective well intervention plans. By analysing well performance data and wellbore integrity, data analytics can identify wells that would benefit most from intervention. Additionally, historical data can provide insights into the success rates and cost-effectiveness of previous well interventions, guiding operators in selecting the most appropriate intervention strategies.
– Predictive analytics for equipment health: In mature oil fields, the reliability of equipment is critical to maintaining optimal production levels. Data analytics can predict equipment health by analysing sensor data from pumps, compressors, and other production equipment. Machine learning algorithms can identify patterns that precede equipment failures, such as vibration patterns or temperature changes. Predictive analytics can alert operators to potential equipment malfunctions, enabling proactive maintenance and preventing costly unplanned downtime.
– Decision support for reinvestment: Data analytics provides essential decision support for reinvestment strategies in mature oil fields. By analysing production data, equipment performance, and reservoir behaviour, operators can evaluate the economic viability of further investment. Data-driven insights can help operators assess the potential returns on investment for well interventions, infrastructure upgrades, or enhanced oil recovery projects. This analysis aids in prioritising investment opportunities based on their expected impact on production and profitability.
Harnessing big data for resource utilisation
The energy industry generates massive volumes of data from various sources, including sensors, equipment, drilling logs, and production records. Big data technologies can efficiently process, store, and analyse this vast amount of information, providing valuable insights to enhance resource utilisation.
Big data analytics can optimise the supply chain by analysing inventory levels, transportation logistics, and supplier performance. By identifying inefficiencies and optimising logistics, the industry can reduce operational costs and enhance overall efficiency.
Enhancing safety and environmental sustainability
Data analytics and big data technologies offer significant benefits in enhancing safety and environmental sustainability in TT. Real-time data analytics can monitor equipment conditions and detect potential safety hazards, enabling proactive maintenance and minimising the risk of accidents.
Environmental sustainability is a growing concern, and the industry faces increasing pressure to reduce its environmental impact. Big data analytics can help monitor emissions, energy consumption, and environmental performance to ensure compliance with environmental regulations. By optimising operations, companies can reduce their carbon footprint, contribute to global sustainability efforts, and enhance their reputation as responsible energy providers.
Overcoming challenges and ensuring data security
While data analytics and big data offer significant opportunities, their implementation in the oil and gas industry of TT also comes with challenges. The industry may face hurdles in managing and integrating disparate data sources, as well as ensuring data quality, accuracy, and consistency. Overcoming these challenges requires significant investments in technology infrastructure, data management systems, and data integration platforms.
Data security is another crucial consideration. The energy industry deals with sensitive information, including proprietary data, exploration findings, and strategic plans. Robust data security measures are essential to protect data from cyber threats and unauthorised access. Companies must adhere to best practices in data governance, encryption, and access controls to safeguard their valuable data assets.
Collaborating and building a data-driven culture
The successful adoption of data analytics and big data technologies in TT's energy industry requires collaboration between industry players, government agencies, and research institutions. Knowledge-sharing and collaboration can facilitate the exchange of best practices, technological expertise, and lessons learned, accelerating the industry's digital transformation.
Furthermore, building a data-driven culture is essential for successful implementation. This cultural shift involves fostering a mindset that values data-driven decision-making, promoting data literacy among the workforce, and investing in data analytics training programs. Emphasising the importance of data-driven insights at all organisational levels can drive greater efficiency, innovation, and continuous improvement.
Data analytics and big data present an unparalleled opportunity for TT’s oil and gas industry to optimise operations, enhance safety, and promote sustainability.
By integrating these technologies into exploration, production, resource utilisation, and environmental management, the industry can navigate challenges and remain competitive in the global energy market. Embracing a collaborative approach and building a data-driven culture will be instrumental in unlocking the full potential of data analytics and big data to propel the oil and gas industry of TT into a sustainable and prosperous future.
This article was submitted by the Geological Society of TT.
Comments
"Using big data, AI to optimise Trinidad and Tobago’s energy sector"