The machine learning challenge
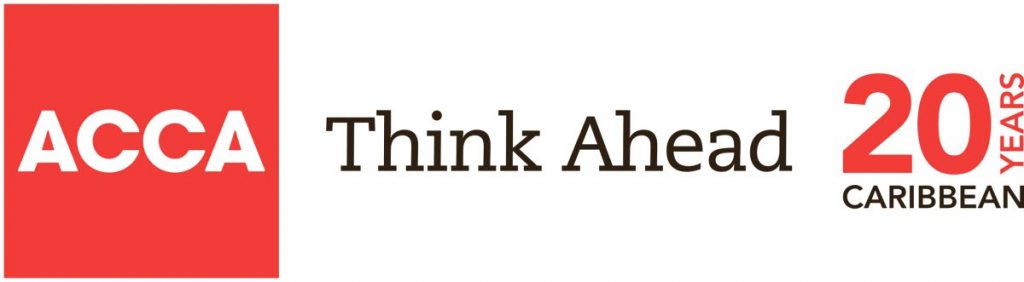
NARAYANAN VAIDYANATHAN, head of business insights, ACCA
Understanding the intricacies of global best practice and adapting to it will set machine learning (ML) on a successful course. Highly skilled accountants are vital to that process.
Issues surrounding ethics and artificial intelligence have risen to prominence in recent years in discussions about artificial intelligence (AI). Across the Atlantic for example, Andrus Ansip, the European Commission’s vice president for digital matters, said in a statement that AI ethics would help people to trust the technology and therefore benefit from it more fully (Fortune, 2019).
ACCA’s recent report, Machine learning: more science than fiction, looks not just at what ML actually is and how it operates, but it also examines the ethical considerations, taking guidance from the fundamental principles established by the International Ethics Standard Board for Accountants (IESBA).
Ethical behaviour and accountability underpins the standard of behaviour expected of a professional accountant and within any organisation it is the key requirement of the finance function to provide tough yet constructive challenges to ensure business decisions are grounded on sound ethical principles.
In seeing ML’s potential, professional accountants need to think of the potential benefits, but also the long-term sustainable advantages and ethical considerations.
Dealing with bias is a frequently discussed source of ethical challenge. ML algorithms, both supervised and unsupervised, need to be fully interpreted in order to avoid for example, confusing correlation with causation. For accountants, objectivity may be compromised and they have to consider whether they have been biased in favour of assuming the outcomes are valid merely because they are supported by an ML algorithm. Consequently, professional scepticism and a mindset willing to take on new challenges are essential to avoid being afraid to dig deeper if they face pressure to ignore the issue.
The most important and non-negotiable requirement for powering the use of ML is data; and organisations must develop a coherent data strategy as meaningful insight with a low likelihood of bias depends on having enough data across all categories. It is this richness of data which enables the algorithm to identify relationships which might not be obvious to a human.
What matters here is professional competence and due care in recognising the potential for breaches. Accountants must understand the increasing risks to data, the regulatory requirements and the financial implications of breaching these. On a practical level, this could mean for example, the ability to identify and assess the controls in place around a data lake or background checks on employees and contracted onto the team. From a risk management standpoint, they may also need to quantify the impact of a data breach on the business and its reputation.
In a complex landscape, business would better confront ethical questions with the help of philosophy. People should not be afraid to make deeper, value judgements. The debate about economics should go beyond technicalities of GDP growth and unemployment, and into deeper considerations of values like freedom, equality, justice, and the nature of society.
An important principle for ethically sound ML solutions is that they should be designed in a way that does not alter the pattern of accountability established by society, culture and law. At the heart of this, who takes responsibility for the consequences of decisions made, the human professional accountant or the algorithm? How this is dealt with will be a key focus of the years ahead. The risk is technology will take the credit when things go well, and humans will take the blame when things go wrong – a no-win situation for those trying to work with the technology. There is accountability for the user of the application, not just its maker. Pertinent to accountants who must consider whether there is sufficient oversight and review of the algorithms’ decisions.
ACCA’s work on emotional quotient (EQ) strongly demonstrated the need in this digital age for competencies related to emotional intelligence - digital quotient (DQ) and EQ are best seen combined for either to be really effective. Accountants can achieve significant improvements in their understanding of ML by questioning the humans associated with developing the model. And not necessarily by becoming experts or understanding complex mathematical algorithms themselves, though a level of comprehension that enables them to engage.
Also, the "black-box" situation where those who developed the model themselves cannot explain how it has arrived at the results is a concern but should not stop professional accountants from continuing to engage as this nascent field starts to increasingly mature. In fact, professional accountants are dealing with challenges in understanding reasons for decisions, in human decision-making as well.
Comments
"The machine learning challenge"